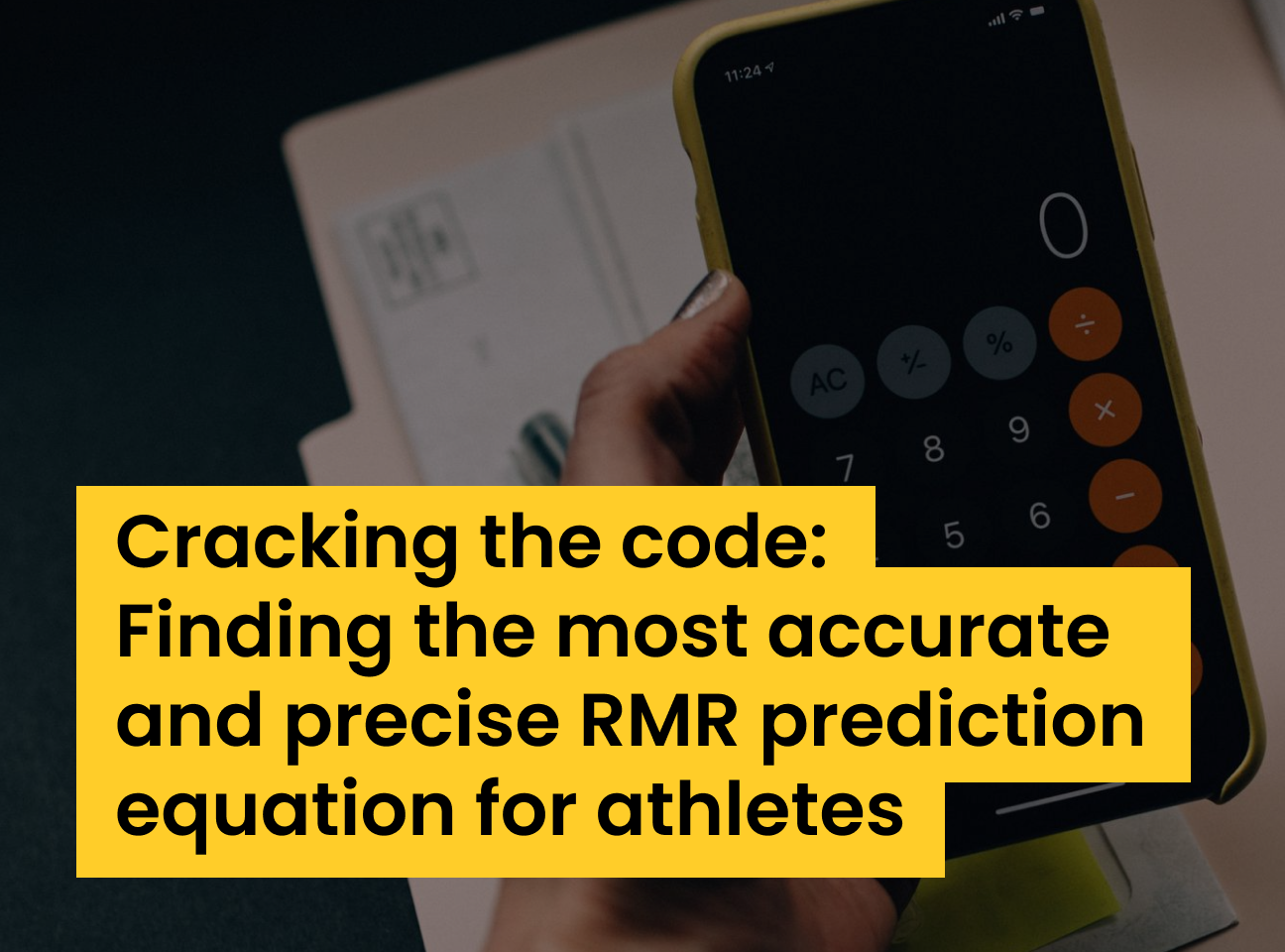
Cracking the code: Finding the most accurate and precise RMR prediction equation for athletes
By Alex Ritson MSc, Head of Education
Research:
Accuracy of Resting Metabolic Rate Prediction Equations in Athletes: A Systematic Review with Meta‑analysis by O’Neill, Corish & Horner (2023)
Introduction:
Resting metabolic rate (RMR) refers to the heat produced by the body at rest. It is the energy expenditure from the billions of ATP-dependent biochemical reactions in the body’s organs and tissues while in a physically inactive state (Heymsfield et al., 2021). It’s like the power a car consumes when in “idle mode” — this is when a car’s engine is running but remains stationary. Even when the vehicle is not running, it still needs to consume fuel to maintain essential functions, such as keeping the battery charged, the engine warm and powering the car’s computer. Laboratory approaches for measuring RMR are direct (heat) or indirect (oxygen and carbon dioxide) calorimetry (Kenny et al., 2017); however, the most practical means for practitioners is to use RMR prediction equations. RMR prediction equations are mathematical formulas used to estimate an individual’s RMR. They are created using a statistical model called regression analysis, which analyses the relationship between a dependent variable and independent variables. In the context of RMR prediction equations, the goal is to use regression analysis to predict the value of the dependent variable (measured RMR via indirect calorimetry) based on independent variables, such as age, weight, height, sex, and body composition of the study population. A prediction equation is developed when researchers find the “best-fitting” equation to the measured RMR of the study population. The best-fitting equation is one that is both accurate (the average predicted values from the equation are closest to the measured values) and precise (minimal variability between participants). For most of us (and many athletes), RMR is the most significant component of total daily energy expenditure, with the exception being athletes with high training/competition demands (think distance runners and triathletes). An accurate prediction of RMR for an athlete is crucial for developing a bespoke nutritional strategy. For instance, even a miscalculation of an athlete’s RMR by ~250 kCal is mathematically equivalent to gaining or losing a kilogram of fat mass over a month. Researchers and practitioners also use RMR prediction equations to assess the energy availability (EA) status of certain athletes (De Souza et al., 2019). A lower ratio of measured RMR to predicted RMR (e.g., 0.90) indicates metabolic suppression in certain athletes (Strock et al., 2020). If we refer back to the idle mode car analogy, low EA is like the battery, engine and computer lowering their output to compensate for a lack of fuel in the tank. Just like how keeping a car in idle mode for too long can cause issues, chronic low EA can have serious health and performance consequences to the athlete. With over 100 RMR prediction equations available, practitioners routinely face the challenge of selecting the best fitting RMR prediction equation for their athletes. This study aimed to address this issue by determining which RMR prediction equations were most accurate and precise for athletes.
The study:
This was a prospectively registered systematic review and meta-analysis, including studies of athletes whereby RMR was measured by indirect calorimetry and compared to RMR prediction equations. The included studies had to report or allow for extrapolation of the accuracy (predicted RMR expressed as a percentage of measured RMR) and precision (percentage/number of participants who fell within the 10% range of measured values) of the studied prediction equations. Subgroup analysis was performed on sex, body composition measurement method, athlete characteristics (athlete status, energy availability, body weight), pre-assessment procedure prior to measurement (e.g., fasting status, caffeine abstinence) and RMR measurement methodology (e.g., RMR discard period).
Findings:
Let’s recap: this systematic review and meta-analysis aimed to determine which RMR prediction equations are most accurate (the average predicted values closest to measured values) and most precise (number of individuals within 10% of measured values) in athletes. The analysis included twenty-nine studies, including 1430 athletes (822 female, 608 male; 34% Tier 1, 10% Tier 2, 49% Tier 3, 7% Tier 4) from various sports. The review found the most accurate (within ±1% of measured RMR) prediction equations to be those “locally derived”. Locally derived RMR equations are those produced from the data collected from study participants. This data includes participant characteristics (age, weight, height, and sex) and their measured RMR. It makes sense for these equations to be the most accurate as they are specific to the study population. However, such equations may not be generalisable to other athlete populations with different characteristics. Eleven equations met the accuracy criteria for the meta-analysis. Five predicted equations: Cunningham (1980), Harris-Benedict (1918), Cunningham (1991), De Lorenzo (1999), and Ten-Haaf (2014) did not differ statistically from measured values in athletes. However, apart from the Ten-Haaf (2014) equation (p=0.48, I² =0%), all other prediction equations demonstrated large heterogeneity (p<0.05, I² range: 80-93%). Put simply, while the group average of predictive values was relatively accurate, there was considerable variability (under-or-over predicting RMR) on an individual level. Only the Ten-Haaf (2014) equation was the exception. Several commonly used equations: Mifflin-St. Jeor (1990), Owen (1988), FAO/WHO/UNU (1985), Nelson (1992), and Koehler DXA (2016) demonstrated significant under-or-over prediction of RMR. Moreover, certain predictive equations showed significant inaccuracies when specific characteristics were considered: sex (Harris-Benedict and Lorenzo), athlete status (Harris-Benedict), or RMR pre-assessment procedures (Cunningham 1980 and Harris-Benedict). Nine studies were included in the analysis for precision. Of the nine assessed, the Ten-Haaf (2014) equation was the most precise, predicting 80.2% of participants to be within ±10% of the measured value, with others ranging from 40.7 to 63.7%. In other words, according to this analysis, using the Ten-Haaf (2014) equation could predict the RMR of 80% of athletes assessed within a 10% range of actual RMR values.
A closer look: What does Tier 1 – 4 mean?
A limitation in sport-science research has been the lack of objective standardisation for categorising participants as it relates to their training status. Qualitative descriptions such as untrained, recreationally active, trained, well-trained, elite, and world-class are often used with minimal uniformity between studies. This can cause problems in the replication of research and its translational potential to practise, as metabolic, physiological, and nutritional responses to exercise are highly dependent on an athlete’s training status. For instance, the ergogenic benefit of beetroot juice/nitrate supplementation has been shown to improve performance primarily in “lower-calibre” athletes, whereas elite athletes do not exhibit the same benefits (Jones et al., 2018). In the context of this research, higher Tier athletes may show higher RMR values due to increased skeletal muscle mass due to genetics and their training demands compared to lower Tier participants. Notably, skeletal muscle mass expends roughly 13.5 kCal/kg compared to 4.5 kCal/kg for adipose tissue (Heymsfield et al., 2021). To circumvent this issue, McKay and colleagues (2022) developed the participant classification framework as a means to provide participant uniformity between research studies. This framework characterises participants into five tiers based on training volume and performance metrics: Tier 0: Sedentary; Tier 1: Recreationally active; Tier 2: Trained/Developmental; Tier 3: Highly Trained/National Level; Tier 4: Elite/International Level; Tier 5: World Class (See Table 1).
Table 1. Participant Classification Framework
Retrieved from McKay et al. (2022)
Relevance
For practitioners without access to indirect calorimetry, finding the best fitting RMR prediction equation for their athlete is critical for developing an effective nutritional strategy. The Harris-Benedict and Cunningham equations have been front-runners for male and female athletes since their recommendation by the Academy of Nutrition and Dietetics, Dietitians of Canada, and the American College of Sports Medicine (Thomas et al., 2016). Moreover, these equations have become standard practice when comparing against measured RMR to discern EA status (Jurov et al., 2022; Strock et al., 2020). This research suggests that we consider a new approach. First, practitioners should consider whether there is a prediction equation that closely matches the characteristics of their athlete (physical, sex, sport, athlete status). If available, the practitioner should go with that equation — for those interested, this paper provides a complete overview of the population used for developing each prediction equation. If not, the practitioner may want to consider whether their athlete’s characteristics fall within those used to derive the Ten-Haaf (2014) equation: Males: 68 kg to 84 kg, and 1.73m and 1.91m in height; females: 54 kg to 70 kg in weight, and 1.67 m and 1.79 m in height. If so, there is an 80% chance the prediction equation will be within <10% of the athlete’s measured RMR. If not, the authors provide guidance on other potential equations to consider (see Figure 1). This new approach of determining the best fitting prediction equation for athletes should also be used when determining the EA status of athletes (e.g., mRMR/pRMR ratio).
Figure 1. A flowchart to guide choice of equation for predicting resting metabolic rate in athletes. Retrieved from O’Neill et al. (2023)
Recommended reading:
Resting Energy Expenditure: From Cellular to Whole‐Body Level, a Mechanistic Historical Perspective by Heymsfield et al. (2021)
Complement this research with the We Do Science podcast episode:
Energy Availability: From Health to Performance with Jose Areta PhD
References:
De Souza, M. J., Koltun, K. J., Strock, N. C., & Williams, N. I. (2019). Rethinking the concept of an energy availability threshold and its role in the Female Athlete Triad. Current Opinion in Physiology, 10, 35–42. https://doi.org/10.1016/j.cophys.2019.04.001
Heymsfield, S. B., Smith, B., Dahle, J., Kennedy, S., Fearnbach, N., Thomas, D. M., Bosy‐Westphal, A., & Müller, M. J. (2021). Resting Energy Expenditure: From Cellular to Whole‐Body Level, a Mechanistic Historical Perspective. Obesity, 29(3), 500–511. https://doi.org/10.1002/oby.23090
Jones, A. M., Thompson, C., Wylie, L. J., & Vanhatalo, A. (2018). Dietary Nitrate and Physical Performance. Annual Review of Nutrition, 38(1), 303–328. https://doi.org/10.1146/annurev-nutr-082117-051622
Jurov, I., Keay, N., & Rauter, S. (2022). Reducing energy availability in male endurance athletes: A randomized trial with a three-step energy reduction. Journal of the International Society of Sports Nutrition, 19(1), 179–195. https://doi.org/10.1080/15502783.2022.2065111
Kenny, G. P., Notley, S. R., & Gagnon, D. (2017). Direct calorimetry: A brief historical review of its use in the study of human metabolism and thermoregulation. European Journal of Applied Physiology, 117(9), 1765–1785. https://doi.org/10.1007/s00421-017-3670-5
McKay, A. K. A., Stellingwerff, T., Smith, E. S., Martin, D. T., Mujika, I., Goosey-Tolfrey, V. L., Sheppard, J., & Burke, L. M. (2022). Defining Training and Performance Caliber: A Participant Classification Framework. International Journal of Sports Physiology and Performance, 17(2), 317–331. https://doi.org/10.1123/ijspp.2021-0451
O’Neill, J. E. R., Corish, C. A., & Horner, K. (2023). Accuracy of Resting Metabolic Rate Prediction Equations in Athletes: A Systematic Review with Meta-analysis. Sports Medicine. https://doi.org/10.1007/s40279-023-01896-z
Strock, N. C. A., Koltun, K. J., Southmayd, E. A., Williams, N. I., & Souza, M. J. D. (2020). Indices of Resting Metabolic Rate Accurately Reflect Energy Deficiency in Exercising Women. International Journal of Sport Nutrition and Exercise Metabolism, 30(1), 14–24. https://doi.org/10.1123/ijsnem.2019-0199
Thomas, Travis. D., Erdman, K. A., & Burke, Louise. M. (2016). Nutrition and Athletic Performance. Medicine & Science in Sports & Exercise, 48(3), 543–568. https://doi.org/10.1249/MSS.0000000000000852